Improved Inception V3 method and its effect on radiologists’ performance of tumor classification with automated breast ultrasound system
Introduction
Breast cancer has surpassed lung cancer as the most commonly diagnosed cancer and the most common cause of death from cancers for women worldwide (1). Mortality can be reduced by early detection and timely therapy. Therefore, it is important to have appropriate methods for screening the earliest signs of breast cancer (2).
Not all breast cancers can be detected by mammography, especially in those occurring in dense breasts (3). We classify breast density according to the proportion of fat and glands contained in breast tissue. Mandelson et al. showed that breast density was strongly associated with reduced mammographic sensitivity (4). Because of more detailed anatomical details of breast tissue than mammography, Ultrasound is widely used as a suitable and valuable complement tool for dense breasts screening. The proportion of women with dense breasts in China is high. In consideration of economic development and the widespread availability of the ultrasound scanning, ultrasound is considered the first-line imaging tool for Chinese women (5).
Conventional ultrasound scanning is performed with a hand-held probe and does not systematically cover the whole breast. It is safe and convenient, but also highly operator-dependent and time-consuming, and records only representative images of the detected lesions that cannot be easily compared with the results of past examinations (6). The automated breast ultrasound (ABUS) was developed to overcome the limitation of operator dependency and lack of standardization and reproducibility of handheld ultrasound (HHUS) (7,8).
The ABUS uses high-frequency sound waves targeted at the breast, covers the entire breast, and the ultrasound scanning process is automated with a motor driving the super-wide probe to cover the whole breast. This imaging technology utilizes ultrasound to scan the entire breast while the patient reclines in a comfortable prone position. There is no breast compression or radiation associated with this ABUS.
Compared to HHUS, ABUS could provide higher quality images due to its standardization of screening and higher frequency sound wave probe. Additionally, it provides three-dimensional (3D) volumetric images of the breast for review by the radiologist. These 3D images are more beneficial to women with dense breasts because they enable radiologists to check the breast from a variety of angles and offer a better interpretation. The generated coronal plane has been suggested to improve diagnostic accuracy. As a newer approach, ABUS could detect up to 30% more cancers in women with dense breast tissue. However, reading ABUS images is time consuming in the current clinical environment. It also requires high skill level for radiologists, which has limited ABUS integration into clinical practice (9-13). Gratifyingly, its standardization of screening made it possible to integrate computer-aided diagnosis (CAD) systems with this new imaging technology.
The CAD systems have been developed as assistant tools for radiologists over several decades. The CAD system comprised a neural network to highlight suspicious areas to assist radiologists in discovering and analyzing the lesions (14). It was initially used to help radiologists by defining a region of interest (ROI) on the mammogram and more recently for the analysis of breast ultrasound images. Previous studies have shown that use of the CAD system on ultrasound could improve the accuracy and efficiency of radiologist diagnoses, but that performance can vary according to the quality of ultrasound images and the type of breast lesions (7,15,16).
As a new detection method which can provide axis plane, coronal plane, and sagittal views, ABUS has received increasing attention of researchers. The coronal plane is formed using the axis plane and provides a new perspective for doctors. The pinprick feature of the coronal plane is the key factor to classify lesions, and researchers have proposed methods of aiding diagnosis of the coronal plane. Tan et al. (17) applied Otsu and a dynamic programming algorithm to achieve segmentation of lesions and then extracted shape features to classify lesions. They reached an accuracy of 0.93 and highlighted that the pinprick feature is the most important feature of malignant tumors. Tan et al. (18) applied the dynamic programming algorithm to segment the coronal plane images of the tumor, extracted textural features of tumors, and used a linear discriminate classifier to diagnose the tumor. This CAD system has helped doctors to improve the diagnostic accuracy from 0.87 to 0.89. Chiang et al. (19) used 3DCNN and hierarchical clustering to detect tumors of coronal plane images and attained a sensitivity of 0.75. In addition, there have been previous studies on axis plane images, including classification and detection. Lo et al. (20) used watershed and logistic regression classification to detect tumors in axis plane images and obtained a sensitivity of 0.8. Drukker et al. (21) utilized k-means and Markov chain Monte Carlo networks to classify tumors and reached a sensitivity of 0.75.
Although existing studies have shown that the use of CAD could reduce the work demands of radiologists, conclusions have been ambiguous regarding the accuracy of breast lesion diagnoses (22,23). Therefore, in our study, we hoped to propose a novel CAD system by extracting the textural features from ABUS images to investigate the efficiency and effectiveness of using CAD for breast cancer detection, and to study the efficiency of the proposed CAD system in helping radiologists to reduce the cost of ABUS interpretation. We also hoped developing this system would help beginners to improve their experience and skill levels. We present the following article in accordance with the STARD reporting checklist (available at https://dx.doi.org/10.21037/gs-21-328).
Methods
Patient selection
All procedures performed in this study involving human participants were in accordance with the Declaration of Helsinki (as revised in 2013). The study was approved by the Ethics committee of Taizhou Hospital of Zhejiang Province (K20201116). Individual consent for this retrospective analysis was waived.
The 3D ABUS system (Invenia ABUS, GE Healthcare, Sunnyvale, CA, USA) was used to collect the ABUS images. The system consists of Invenia ABUS Scan Station with wide field-of-view transducer (Reverse CurveTM ultra-broadband transducer, GE Healthcare; frequency, 6–15 MHz; aperture length, 15.4 cm; transducer bandwidth, 85%; imaging depth, up to 5.0 cm) and Invenia ABUS workstation.
From May 2018 to October 2018, a total of 368 women were examined with ABUS in the Zhejiang Taizhou Hospital. The results revealed 84 healthy women, 101 womenwith benign lesions with years of negative follow-up, and 183 women with 205 lesions advised to pursue biopsy or surgical treatment. Of the 183 women, all those recruited had at least 1 palpable mass; while non-mass breast lesions and lesions without histological confirmation were excluded from the study group. Finally, 149 breast nodules in 135 participants were included in this study. The flowchart in Figure 1 further clarifies our study design.
Mean age for all participants was 51.05±10.549 years (range, 23–83 years), mean size for all lesions was 18.89±10.238 mm (range, 5–59 mm).The patients and nodules characteristics are shown in Table 1.
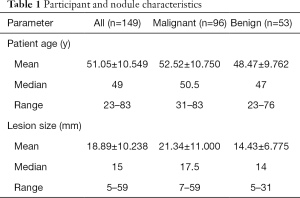
Full table
II3 method
Tumor segmentation
Tumor segmentation is an essential step for the extraction of tumor features. A fully automatic BUS image segmentation approach based on breast characteristics in space and frequency domains was utilized to locate the ROIs and acquire the tumor contours (24). First, an adaptive reference point (RP) generation algorithm was utilized which can produce the RPs automatically based on the breast anatomy. Further, a multipath search algorithm was proposed to generate the seeds quickly and accurately. In the tumor segmentation step, a segmentation framework was proposed in which the cost function was defined in terms of tumor boundary and region information in both frequency and space domains. The frequency constraint was firstly built based on an edge detector which was invariant to contrast and brightness; and then the tumor shape, position, and intensity distribution were modeled to constrain the segmentation in the spatial domain. The well-designed cost function was graph-representable and its global optimum was locatable.
Tumor feature extraction
Research has found that image textures are useful for ultrasound image classification and tumor recognition (25-27). Gray level co-occurrence matrix (GLCM) is a second-order histogram for describing image textures which can be represented as a symmetric matrix to quantify the joint distribution of pairwise pixels. The GLCM depicts the spatial distribution of the pixel. Based on the GLCM, in this study we extracted textural features of the tumor from the image, which included energy, correlation, homogeneity, mean, and variance.
Mean was the average value of the region and reflected the brightness information of the region. The calculation formula of the mean value was as follows, where P is the grayscale co-occurrence matrix, M and N are the number of rows and columns of the grayscale co-occurrence matrix.
The SD of the corresponding region of the GLCM reflected the change in degree and uniformity of the gray level of the region. The formula for calculating the SD was as follows.
Roughness described the smoothness of the tumor boundary. The formula was as follows.
Homogeneity represented a measure of the local grayscale uniformity of the image. The formula was as follows:
Energy described changes of texture at different positions in the image. The calculation formula of energy was as follows.
Entropy reflected the randomness of the distribution of the gray value in the tumor region. When the distribution of the gray value in the tumor region was complicated, the entropy was larger. The formula for calculating entropy was as follows.
Correlation described the linear dependence between pixel values on an image, which was the description of the approximation of the region’s gray level in a certain direction. The greater the approximation, the greater the value. The formula for correlation was as follows.
Shape features are the description of the appearance. According to the shape feature extraction algorithm, this article extracted shape features of the tumor of the ABUS images: roundness, normalization aspect ratio, average normalized radial length, normalized radial length standard deviation, entropy of the average normalized radial length, area ratio, aspect ratio, number of lobular, needle shape, boundary roughness, direction angle, elliptic normalized circumference and oval normalized contour.
(I) Roundness: when the shape of the tumor area is close to a circle, its roundness is close to 4II. Roundness was defined as follows, where P represents the perimeter of the tumor area and A represents the site of the tumor.
(II) Normalization aspect ratio describes the aspect ratio of the external matrix of the closed area of the tumor. Aspect ratio is a measure of tumor shape, and the closer the aspect ratio is to 1, the more likely the tumor is to be benign.
(III) Average normalized radial length: the center of the tumor and the edge of the area were used in this calculation. Calculating each boundary point to the center of the tumor area is the radial length. Then the distance was normalized and each radial length divided by the maximum radial length was the normalized radial length. And the mean of the normalized length was the average normalized radial length. The formula to calculate average normalized length was as follows, where (xi,yi) is the coordinate of the its boundary point and (x0,y0) is the coordinate of the region center.
(IV) Normalized radial length standard deviation: this described the distribution of normalized radial length of the tumor and reflected the aggregation degree of normalized radial length. The calculation formula was as follows, where is the average normalized radial length.
(V) Entropy of mean normalized radial length: we divided the maximum and minimum of the normalized radial lengths of boundary points into 100 parts, then conducted probability statistics of length values, and calculated the corresponding probability values of all lengths. The formula for calculating the entropy of normalized radial length was as follows, where p is the corresponding probability of occurrence of all lengths.
(VI) The calculation formula of area ratio was as follows, where A is the sum of the minus between the distance greater than the average normalized radial length and the average normalized radial length, davg is the average normalized radial length, and circum is the number of edge points in the tumor region.
(VII) The calculation formula of aspect ratio is as follows, where maxD is equal to the maximum normalized length of all edge points, and minD is the minimum normalized radial length of all edge points.
(VIII) Number of lobules refers to the lobules of the tumor. A benign tumor usually has the growth pattern of a single leaf, while a malignant tumor generally presents the growth pattern of multiple leaves. The calculation formula was as follows: Posminnum is the minimum number of the fitting curve, and posmaxnum is the maximum number of the fitting curve.
(IX) Needle-like degree is an important indicator for judging the benign tumors from the malignant ones. Typically, the boundary of malignant tumor is burry, while that of benign tumor is smooth. The calculation formula of needle-like degree was as follows, where f1 is the accumulation of the value after the Fourier transform of the corresponding length when the angle value of the polar coordinates of the edge point is less than , and f2 is the accumulation of the value after the Fourier transform of the corresponding length when the angle value of the polar coordinates of the edge point is greater than and less than II.
(X) Boundary roughness was the sum of the difference between the radius value of each boundary point in polar coordinates and the value of its adjacent points. The boundary roughness (rough) was defined as.
where cricum is the number of boundary points
(XI) Direction angle was the radian value of each closed area. The calculation formula was as follows, where orientation is the angle value of the closed region.
(XII) The formula of the normalized perimeter of the ellipse was as follows. ShortAxis represents the length of the short axis of the ellipse while LongAxis represents the length of the long axis of the ellipse.
(XIII) The formula of the elliptic normalized contour was as follows, where skeletonum is the sum of the number of points with the shortest distance from each row of the tumor region to the boundary.
Tumor classification
A total of 149 images were collected, including 96 malignant images and 53 benign images. Benign and malignant images were divided into five groups, with the number of benign images in each group being 11, 11, 11, 10, 10 and the number of malignant images in each group being 19, 19, 19, 19, 20. In the experiment, a group of benign images and a group of malignant images were combined as a test set, and the remaining 4 groups of benign and four groups of malignant images were used as a training set. The training set was input into each model for learning, and the average classification performance was obtained by using the test set.
Support vector machine (SVM) and logistic regression
As a traditional classification algorithm, SVM is based on the principle of maximizing the diversity among classes. For the linear case, it is preferable to find a line so that the samples on both sides of the line are maximally distanced from the line. The equation of the line was as follows, where represents the weight of the sample attribute and represents the sample attribute.
The hypothesis model of logistic regression was as follows, where X is the sample input, hӨ(X)is the output of the model, and Ө is the model parameter of the required solution.
where
Inception V3 network
Inception V3 consists of 47 layers. We used 2 convolution kernels of 3*3 to replace 5*5 convolution kernels, and 2 convolution kernels of 3*1 and 1*3 to replace 3*3 convolution kernels to reduce the training network parameters and ensure the classification performance of the network. The fully joined convolutional layer were converted to the coefficient joint layer for reducing the computation. Batch normalization was utilized to solve the problem of gradient disappearance. The network structure is shown in Figure 2.
The method proposed in this paper
The proposed approach integrated SVM, logistic regression, and Inception V3 network classifiers, with a voting mechanism for final classification.
Methods and doctors’ diagnosis
We assigned 3 novice readers with less than 3 years of experience in breast ultrasound image interpretation and 3 experienced readers with more than 10 years in breast ultrasound image interpretation, who were blinded to the patients’ information, to review the imaging data and differentiate between malignant and benign among the 149 breast nodules.
After the readers completed full unaided diagnoses, the proposed CAD was utilized to detect and analyze the same breast images and generate a list indicating which nodules were malignant and which were benign.
With the proposed CAD system, the 3 novice readers and 3 experienced readers were assigned to re-review the imaging data.
The reading steps are shown in Figure 3.
Evaluation methods
To evaluate the overall performance of each diagnostic system, five descriptive statistics [accuracy (ACC), sensitivity (SEN), specificity (SPE), positive predictive value (PPV), and negative predictive value (NPV)] were used. The number of correctly and incorrectly classified malignant tumors were defined as true positive (TP) and false negative (FN), and the number of correctly and incorrectly classified benign tumors were defined as true negative (TN) and false positive (FP). The 5 descriptive statistics are calculated as:
Another criterion for evaluating the performance of the classifier system was the receiver operator characteristic curve (ROC). The curves were constructed by computing the sensitivity and specificity of increasing numbers of clinical findings in the predicting step. The most commonly used global index of diagnostic accuracy is the area under the ROC curve (AUC). Values of AUC close to 1.0 indicated that the marker had a high diagnostic accuracy; otherwise, the performance was deemed low.
Statistical analysis
IBM SPSS Statistics 22.0 for statistical analyses were used in this study. The SEN, SPE, ACC, PPV, and NPV of each modality were calculated. Student’s t-test and χ2 test were used and P values were calculated. Receiver operating characteristic (ROC) curve was performed to analyze the sensitivity and specificity of different systems of CAD. Macro-average ROC curve was performed to analyze the sensitivity and specificity of different methods of reading ultrasound images.
Results
Pathologic results
In total, 149 breast nodules were studied, consisting of 96 (64.4%) malignant cases identified via biopsies or surgery and 53 (35.6%) benign cases. The malignant lesion-subset included 71 invasive ductal carcinoma, 11 ductal carcinoma in situ, 4 invasive mucinous carcinoma, 3 invasive lobular carcinoma, 1 encapsulated papillary carcinoma, and 6 other malignant lesions. The benign lesion subset consisted of 32 fibroadenomas, 9 adenosis, 8 intraductal papilloma, 3 inflammation of breast, and 1 benign phyllodes tumor. More pathologic results are summarized in Table 2.
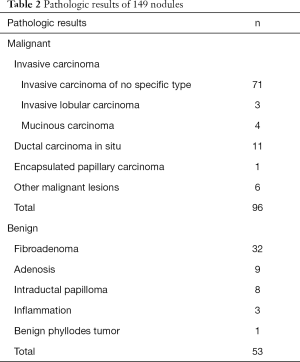
Full table
Results of doctors’ diagnosis
Statistical analysis
The SEN, SPE, ACC, PPV, and NPV of each modality were record as shown in Table 3.
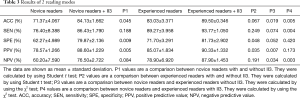
Full table
As can be seen from Table 3 above, the 3 novice readers had an average accuracy of 71.37%±4.067% on the first reading while the 3 experienced readers had an average accuracy of 83.03%±3.371% on the first reading. The difference between the two groups was statistically significant (P=0.019<0.05). Experience was likely one of the most important factors affecting the diagnostic results between the two groups.
With the help of II3 on the second reading, the average accuracy of the novice readers increased to 84.13%±1.662% and that of the experienced readers increased to 89.50%±0.346%. The difference between the two groups was statistically significant (P=0.005<0.05).
In addition, ROC curves were constructed and the AUCs were used as the criterion for diagnostic accuracy. Values of AUC close to 1.0 indicated that the method had high diagnostic accuracy. As displayed in Figure 4A,B, the mean AUC of the novice readers was improved from 0.7751 (without II3) to 0.8232 (with II3). The mean AUC of the experienced readers was improved from 0.8939 (without II3) to 0.9211 (with II3). From Figure 4C, we found the mean AUC for all readers improved in both the second-reading mode (from 0.8345 to 0.8722, P=0.0081<0.05).
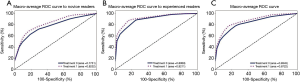
Diagnostic error analysis
Among the 149 breast nodules, there were 77 cases that were misidentified by novice readers; for each misidentified case, at least 1 novice reader made the same mistake. Meanwhile, experienced readers misjudged 41 cases, and for each misjudged case, there was at least 1 experienced readers who made the mistake.
The diagnostic errors made by these readers during the first reading were mainly via the following aspects:
First, they might have been misled by atypical ultrasound features of breast nodules, such as malignant nodules with oval shapes or benign nodules with indistinct margins. In this study, about 47 (47/77, 61.0%) cases were misjudged by the novice readers, compared with 21 (21/41, 51.2%) misjudged by the experienced readers.
Second, the presence of calcifications was a key factor affecting diagnostic accuracy.
Calcifications or small deposits of calcium are common in the breast. Breast calcifications are not always but can be a sign of early breast cancer. The calcifications associated with breast cancer are usually quite small, often <2 mm, and are described as micro-calcifications. Micro-calcifications are among the earliest signs of a breast carcinoma.
Ultrasound does not have an advantage in detecting micro-calcifications of early breast cancer, due to its high false positive rate and low specificity. It also confused the judgment of the novice readers more than the experienced readers. Our study showed that about 13 (13/77, 16.9%) cases in which calcifications were found in nodules were misjudged by the novice readers, compared with 5 (5/41, 12.2%) cases judged the same way by experienced readers.
Furthermore, whether novice or experienced, the mass’s maximum diameter was another key factor that interfered with the readers’ interpretations. The smaller a breast mass is, the more difficult for the radiologist to analyze the lesion; especially, if the maximum diameter of the mass is smaller than 1 cm. About 8 (8/77, 10.4%) cases were misjudged by the novice readers and 5 (5/41, 12.2%) cases by the experienced readers, the difference between two groups was not significant.
Typical error cases for example are in Figures 5,6,7,8.
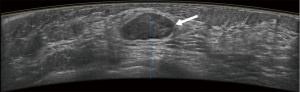
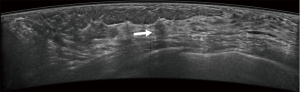
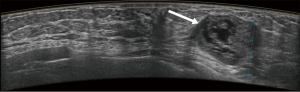
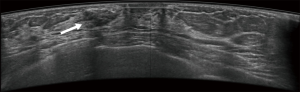
Results of CAD system
Features extraction
The GLCM was utilized for extracting 51 gray features of tumors from 149 images. The extracted features included the energy, correlation, contrast, and homogeneity characteristics at 4 different directions and 3 different distances, as well as the mean variance and entropy of the tumor. Shape features extraction algorithm was performed to extract the 13 shape features including roundness, aspect ratio, average normalized radial length, normalized radial length standard deviation, entropy of the average normalized radial length, area ratio, aspect ratio, number of lobular, needle shape, boundary roughness, direction angle, elliptic, and normalized circumference oval normalized contour. The results of feature extraction are shown in Figure 9. It can be seen that homogeneity in the textural features had a significant degree of differentiation in the judgment of benign and malignant tumors in ABUS images. Among the shape features, the needle-like degree had the greatest differentiation for the benign and malignant tumors (Figure 9B).
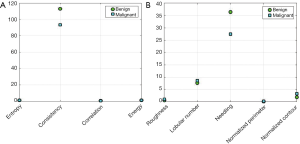
Benign and malignant classification
In this study, SVM and logistic regression were utilized to construct the classification model because of its high generalization performance without need to add a priori knowledge and ease of explanation. We used 5-fold cross-validation to ensure that there was no leakage of information between the training and test phases of SVM training and testing.
The tumor characteristics of the training set were input into the SVM and logistic regression classifier to construct the model, and the test set was used for testing. The classifiers with extracted image features as inputs were performed on training folds only and then applied to the corresponding test fold. All image data of a given participant were either all in a training fold or all in a test fold. The average results obtained from the method by using 5-cross validation are shown in Table 4.
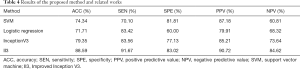
Full table
The training image set was input into the Inception V3 network for feature extraction and classification, and then tested using the validation set. The ROC curves of 3 three methods are shown in Figure 10.
Discussion
The proposed CAD can highlight the areas of suspicious findings to assist radiologists in discovering and analyzing lesions. Several studies have shown the potential of CAD in image-recognition tasks and demonstrated that CAD is capable of detecting breast cancers which had been dismissed by radiologists (28).
Previous studies have revealed that the performance of CAD systems can vary according to CAD itself, the quality of ultrasound images, and the type of breast lesions. Most previous studies on the application of CAD have focused on the recognition of breast nodules using B-mode ultrasound. In this study, a novel CAD system was proposed based on ABUS images. This CAD system integrated SVM, logistic regression, and Inception V3 network classifiers, with a final result of 88.6% accuracy.
We found that the novel CAD system can accurately locate the tumor, whether or not the tumor is in a specific location, is large, small, or poorly differentiated from the background. In addition, the proposed method can help doctors, particularly in the task of reading cases which have atypical shape and edge characteristics. We studied the value features extracted by the proposed method. Homogeneity, as defined in Eq. [4] is an important feature to describe the local texture of image. A higher value indicated a more irregular local texture. The average of homogeneity for benign tumors was 94.33, and 74.99 for benign tumors.
Our data showed that by using the proposed CAD diagnosis as the reference for re-analysis, the range of improvement was statistically significant for the novice readers (from 71.37%±4.067% to 84.13%±1.662%, P=0.045<0.05), and was not statistically significant for the experienced readers (from 83.03%±3.371% to 89.50%±0.346%, P=0.067>0.05). This meant that the diagnostic accuracy of both groups had been improved with the help of II3, and II3 was more helpful for novice readers than experienced readers. Previous studies have shown that less experienced readers benefit more from CAD than experienced readers. Balleyguier et al. showed that the use of CAD is more helpful for junior radiologists (29), which was consistent with the findings in this study. Meanwhile, our results showed that II3 can not completely compensate for and replace the influence of experience in the diagnostic process. At present, it is recommended that CAD system continues to play an auxiliary role in the clinic.
Additionally, with the help of II3, the diagnostic specificity of the novice readers increased from 62.27%±4.989% to 79.87%±2.136% (P=0.009<0.05), and that of the experienced readers increased from 71.70%±3.291% to 81.73%±2.902% (P=0.048<0.05). The higher the sensitivity, the lower the missed diagnosis rate; the higher the specificity, the lower the misdiagnosis rate. These results indicated that the main benefit of II3 is mainly reflected in reducing misdiagnosis.
The value of CAD is not only in the differentiation of benign and malignant breast nodules, but it can also enhance the experience and skill of some beginners. By using this novel CAD system, the diagnostic skill of beginners can be raised to that of experienced doctors and the diagnosis level of senior doctors can be further improved.
Conclusions
In this paper, we proposed a novel CAD system based on ABUS images, which integrated the SVM, logistic regression, and Inception V3 network classifiers [Improved Inception V3 (II3)].
Rather than conventional ultrasound, in this study, ABUS imaging was selected for its high-quality images. Compared to handheld ultrasound, ABUS imaging can provide clearer and complete information of breast nodules because of its higher frequency sound wave probe and its standardization screening.
This paper extracted a series of textural features of the tumor from the image including energy, correlation, homogeneity, mean, and variance. Through the experiment, we found that homogeneity is an important feature for describing the local texture of images. A higher value indicated a more irregular local texture. The average homogeneity for benign tumors was 94.33, and 74.99 for benign tumors.
The final accuracy of the proposed system was 88.6%, which was superior to the average accuracy of the experienced reader.
Meanwhile, with the help of II3, the average accuracy of novice reader was increased from 71.37%±4.067% to 84.13%±1.662% and the experienced readers increased from 83.03%±3.371% to 89.50%±0.346%, which demonstrated that less experienced readers would benefit more from CAD than experienced readers.
Finally, this study had several limitations. First, it was a retrospective study. The datasets used in this study were pathologically confirmed benign and malignant cases, in which normal cases and benign lesions without surgical treatment were excluded. Therefore, selection and recall biases were unavoidable. Second, sample capacity was small and the algorithm was based on the material collected. We will extend our dataset in the future as we believe that the overall performance of the algorithm will be improved by the inclusion of a larger dataset.
Acknowledgments
Funding: None.
Footnote
Reporting Checklist: The authors have completed the STARD reporting checklist. Available at https://dx.doi.org/10.21037/gs-21-328
Data Sharing Statement: Available at https://dx.doi.org/10.21037/gs-21-328
Conflicts of Interest: All authors have completed the ICMJE uniform disclosure form (available at https://dx.doi.org/10.21037/gs-21-328). The authors have no conflicts of interest to declare.
Ethical Statement: The authors are accountable for all aspects of the work in ensuring that questions related to the accuracy or integrity of any part of the work are appropriately investigated and resolved. All procedures performed in this study involving human participants were in accordance with the Declaration of Helsinki (as revised in 2013). The study was approved by the Ethics committee of Taizhou Hospital of Zhejiang Province (K20201116). Individual consent for this retrospective analysis was waived.
Open Access Statement: This is an Open Access article distributed in accordance with the Creative Commons Attribution-NonCommercial-NoDerivs 4.0 International License (CC BY-NC-ND 4.0), which permits the non-commercial replication and distribution of the article with the strict proviso that no changes or edits are made and the original work is properly cited (including links to both the formal publication through the relevant DOI and the license). See: https://creativecommons.org/licenses/by-nc-nd/4.0/.
References
- Sung H, Ferlay J, Siegel RL, et al. Global Cancer Statistics 2020: GLOBOCAN Estimates of Incidence and Mortality Worldwide for 36 Cancers in 185 Countries. CA Cancer J Clin 2021;71:209-49. [Crossref] [PubMed]
- Tarique M. Fourier Transform Based Early Detection of Breast Cancer by Mammogram Image Processing. J Biomed Eng Med Imaging 2015;2:17-31. [Crossref]
- Zeng H, Veeramootoo JS, Ma G, et al. Clinical value and feasibility of ISET in detecting circulating tumor cells in early breast cancer. Transl Cancer Res 2020;9:4297-305. [Crossref]
- Mandelson MT, Oestreicher N, Porter PL, et al. Breast density as a predictor of mammographic detection: comparison of interval- and screen-detected cancers. J Natl Cancer Inst 2000;92:1081-7. [Crossref] [PubMed]
- Wu GG, Zhou LQ, Xu JW, et al. Artificial intelligence in breast ultrasound. World J Radiol 2019;11:19-26. [Crossref] [PubMed]
- Agarwal R, Diaz O, Lladó X, et al. Lesion Segmentation in Automated 3D Breast Ultrasound: Volumetric Analysis. Ultrason Imaging 2018;40:97-112. [Crossref] [PubMed]
- Tan T, Platel B, Huisman H, et al. Computer-aided lesion diagnosis in automated 3-D breast ultrasound using coronal spiculation. IEEE Trans Med Imaging 2012;31:1034-42. [Crossref] [PubMed]
- Li JW, Li N, Jiang YZ, et al. Ultrasonographic appearance of triple-negative invasive breast carcinoma is associated with novel molecular subtypes based on transcriptomic analysis. Ann Transl Med 2020;8:435. [Crossref] [PubMed]
- Lin X, Jia M, Zhou X, et al. The diagnostic performance of automated versus handheld breast ultrasound and mammography in symptomatic outpatient women: a multicenter, cross-sectional study in China. Eur Radiol 2021;31:947-57. [Crossref] [PubMed]
- Girometti R, Tomkova L, Cereser L, et al. Breast cancer staging: Combined digital breast tomosynthesis and automated breast ultrasound versus magnetic resonance imaging. Eur J Radiol 2018;107:188-95. [Crossref] [PubMed]
- Kaplan SS. Automated whole breast ultrasound. Radiol Clin North Am 2014;52:539-46. [Crossref] [PubMed]
- Zanotel M, Bednarova I, Londero V, et al. Automated breast ultrasound: basic principles and emerging clinical applications. Radiol Med 2018;123:1-12. [Crossref] [PubMed]
- Skaane P, Gullien R, Eben EB, et al. Interpretation of automated breast ultrasound (ABUS) with and without knowledge of mammography: a reader performance study. Acta Radiol 2015;56:404-12. [Crossref] [PubMed]
- Dromain C, Boyer B, Ferré R, et al. Computed-aided diagnosis (CAD) in the detection of breast cancer. Eur J Radiol 2013;82:417-23. [Crossref] [PubMed]
- Shan J, Alam SK, Garra B, et al. Computer-Aided Diagnosis for Breast Ultrasound Using Computerized BI-RADS Features and Machine Learning Methods. Ultrasound Med Biol 2016;42:980-8. [Crossref] [PubMed]
- Ding J, Cheng HD, Min X, et al. Local-weighted Citation-kNN algorithm for breast ultrasound image classification. Optik 2015;126:5188-93. [Crossref]
- Tan T, Platel B, Twellmann T, et al. Evaluation of the effect of computer-aided classification of benign and malignant lesions on reader performance in automated three-dimensional breast ultrasound. Acad Radiol 2013;20:1381-8. [Crossref] [PubMed]
- Tan T, Platel B, Mus R, et al. Computer-aided detection of cancer in automated 3-D breast ultrasound. IEEE Trans Med Imaging 2013;32:1698-706. [Crossref] [PubMed]
- Chiang TC, Huang YS, Chen RT, et al. Tumor Detection in Automated Breast Ultrasound Using 3-D CNN and Prioritized Candidate Aggregation. IEEE Trans Med Imaging 2019;38:240-9. [Crossref] [PubMed]
- Lo CM, Chen RT, Chang YC, et al. Multi-dimensional tumor detection in automated whole breast ultrasound using topographic watershed. IEEE Trans Med Imaging 2014;33:1503-11. [Crossref] [PubMed]
- Drukker K, Sennett CA, Giger ML. Computerized detection of breast cancer on automated breast ultrasound imaging of women with dense breasts. Med Phys 2014;41:012901 [Crossref] [PubMed]
- Sahiner B, Chan HP, Roubidoux MA, et al. Malignant and benign breast masses on 3D US volumetric images: effect of computer-aided diagnosis on radiologist accuracy. Radiology 2007;242:716-24. [Crossref] [PubMed]
- Yang S, Gao X, Liu L, et al. Performance and Reading Time of Automated Breast US with or without Computer-aided Detection. Radiology 2019;292:540-9. [Crossref] [PubMed]
- Xian M, Zhang Y, Cheng HD. Fully automatic segmentation of breast ultrasound images based on breast characteristics in space and frequency domains. Pattern Recogn 2015;48:485-97. [Crossref]
- Huang Q, Huang Y, Luo Y, et al. Segmentation of breast ultrasound image with semantic classification of superpixels. Med Image Anal 2020;61:101657 [Crossref] [PubMed]
- Xian M, Zhang Y, Cheng HD, et al. Automatic breast ultrasound image segmentation: A survey. Pattern Recogn 2018;79:340-55. [Crossref]
- Cheng HD, Cai X, Chen X, et al. Computer-aided detection and classification of microcalcifications in mammograms: a survey. Pattern Recogn 2003;36:2967-91. [Crossref]
- Henriksen EL, Carlsen JF, Vejborg IM, et al. The efficacy of using computer-aided detection (CAD) for detection of breast cancer in mammography screening: a systematic review. Acta Radiol 2019;60:13-8. [Crossref] [PubMed]
- Balleyguier C, Kinkel K, Fermanian J, et al. Computer-aided detection (CAD) in mammography: does it help the junior or the senior radiologist? Eur J Radiol 2005;54:90-6. [Crossref] [PubMed]
(English Language Editor: J. Jones)