Identification of m6A-related lncRNAs for thyroid cancer recurrence
Highlight box
Key findings
• Eight m6A-related lncRNAs risk models were constructed, which play an important role in predicting PFS in patients with recurrent THCA.
What is known and what is new?
• Some studies have shown that m6A-lncRNA can be used as a biomarker for the prognosis and immunotherapy of pancreatic cancer.
• We identified m6A-lncRNA associated with THCA recurrence and constructed a risk model to predict PFS in patients with THCA recurrence.
What is the implication, and what should change now?
• The expression level of m6A-related lncRNA can be used as a basis to judge the recurrence of THCA patients, and timely attention will help to find bad prognosis as early as possible.
Introduction
Thyroid cancer (THCA) is the most common type of endocrine cancer, and its incidence is steadily increasing. THCA has a generally good prognosis, with a 98.1% 5-year survival rate (1). However, patients with stage IV only have a 50% 5-year survival rate, and the recurrence rate is as high as 20% (2). THCA can be divided into 4 subtypes: papillary thyroid cancer (PTC), follicular thyroid cancer (FTC), anaplastic thyroid cancer (ATC), and medullary thyroid cancer (MTC). PTC, which accounts for 75–85% of THCA, is curable but can become more aggressive and lethal when dedifferentiation and metastasis to the cervical lymph nodes occur. FTC is also a differentiated subtype of THCA and involves capsular or vascular invasion. In contrast, ATC is an aggressive undifferentiated tumor with an extremely poor prognosis, contributing to 50% of deaths from THCA. Most cases of MTC are sporadic and usually diagnosed at stage III or IV with nodal metastasis, and MTC patients often show poor prognosis (3). The causes of recurrence are related to a number of factors, including older age, larger tumors, metastases in the cervical lymph nodes, extrathyroidal extension, and lymphatic infiltration (4), and lifelong monitoring of recurrence is required after treatment. For this reason, some consider recurrence to be a more appropriate prognostic endpoint for THCA (5).
The accumulation of epigenetic alterations is important for promoting the development of tumors. Long noncoding RNAs (lncRNAs), which are aberrantly expressed in cancers, have been found to have a central role in epigenetic regulation and been identified as novel biomarkers for early diagnosis and treatment (6,7). LncRNA, a type of functional noncoding RNA transcript that is longer than 200 nt in length, is implicated in a wide range of biological processes in tumor cells, including supporting proliferative signaling, evasion of immune destruction and surveillance, acquisition of replicative immortality, induction of angiogenesis, and activation of invasion and metastasis (8-10). LncRNA SNHG15 has been found to have a role in the proliferation, migration, and invasion of THCA and is related to age, clinicopathological features, and disease-free survival (11).
N6-methyladenosine (m6A) methylation modifications are the most frequent internal RNA modifications in eukaryotes (12). A growing number of findings suggest that noncoding RNA level may be associated with m6A methylation modifications (13). For example, researchers have found that lncRNA XIST can form the RBM15/RBM15B-WTAP-METTL3 complex to regulate transcriptional silencing of genes, which recruits the silencing complex (14). In bladder cancer, METTL3 increases the m6A level of CDCP1 and promotes its YTHDF1-regulated translation (15), leading to tumor cell proliferation, migration, and invasion. In lung adenocarcinoma, METTL3 can catalyze m6A and participate in the postmethylation regulation of target messenger RNA (mRNA), thereby acting as a reader and causing tumorigenesis (16). Yu et al. constructed a m6A-lncRNA signature and nomogram with bioinformatic methods to determine its relationship with immune cells and the microenvironment, confirming that m6A-lncRNA could be used as a biomarker for pancreatic cancer prognosis and immunotherapy (17). However, it is unknown whether the m6A modification of hub lncRNAs (m6A-lncRNAs) is associated with prognostic recurrence in THCA.
In the current study, we aimed to identify m6A-lncRNAs in THCA recurrence and explore the biological features and prognostic value for providing a potential therapeutic approach to predict recurrence of THCA. We present the following article in accordance with the TRIPOD reporting checklist (available at https://gs.amegroups.com/article/view/10.21037/gs-22-678/rc).
Methods
Data acquisition and differentially expressed lncRNAs (DELs)
RNA sequencing (RNA-seq) expression data and clinical characteristics of THCA were obtained from The Cancer Genome Atlas (TCGA) database (https://portal.gdc.cancer.gov/), which included 568 samples (510 tumors and 58 normal tissues). There were 108 out of 510 THCA samples containing clinical information, including 46 recurrent samples and 62 nonrecurrent samples. The DESeq2 package in R was used to analyze THCA samples versus normal samples. Log2fold change >1.0 and P value <0.05 were utilized to recognize DELs. The DELs associated with THCA recurrence were analyzed in recurrent and nonrecurrent THCA samples with the limma R package (version 4.1.1) with an adjusted false discovery rate (FDR) of P<0.05.
Collection of clinical samples
The study included patients who underwent surgical resection of THCA at the First Affiliated Hospital of Jiamusi University. Recurrent and nonrecurrent THCA specimens obtained from the enrolled patients were analyzed by real-time quantitative polymerase chain reaction (RT-qPCR). Two pathologists made the pathological diagnosis. All tumors were diagnosed and classified through histological section and thyroid sonography according to the American Thyroid Association (ATA). The study was conducted in accordance with the Declaration of Helsinki (as revised in 2013). The study was approved by Medical Ethics Committee of the First Affiliated Hospital of Jiamusi University (No. 2020023) and informed consent was taken from all the patients.
Correlation analysis of lncRNAs and m6A-related genes
The association between 23 m6A-related genes and lncRNAs was assessed by Pearson analysis using the corrplot package in R (version 0.92). LncRNAs with P value <0.05 were selected as m6A-related lncRNAs. A m6A-lncRNA coexpression network was built for THCA recurrence according to the Pearson correlation coefficient (PCC) determined by Pearson analysis. To construct the coexpression network, we selected dysregulated m6A-lncRNA pairs with an absolute PCC ≥0.3 and P value <0.05.
Bioinformatics analysis
On the basis of the expression of m6A-related recurrent lncRNAs in THCA samples from the TCGA database, we performed k-means clustering analysis. Additionally, we performed correlation analysis of the clinical characteristics. In calculating the infiltration of the 22 types of immune cells in thyroid tumors, the CIBERSORT algorithm (https://cibersort.stanford.edu/) was applied. If the P value of the inferred immune cell population was less than 0.05, it was considered accurate. The ESTIMATE package in R was used to assess differences in the tumor microenvironment among the 3 clusters. Gene set enrichment analysis (GSEA) was performed on the genes to determine which candidate pathways were activated or inhibited. We performed GSEA analysis for 3 THCA subtypes in two pairs and selected pathways with FDR <0.05 and P<0.05. Kaplan-Meier survival analysis was done to analyze differences in progression-free survival (PFS) between the high-risk group and low-risk group with the R package survminer and the survival tool.
Development of recurrence-related lncRNA prognostic model
To evaluate the prognostic value of the recurrence-associated lncRNAs, we performed Cox regression analysis to investigate the relationships between each lncRNA and survival status in the TCGA cohort. In order to avoid omissions, we used a cut-off P value of 0.05 and found 8 recurrence-related lncRNAs to build the predictive model. The risk score was derived once the TCGA expression data had been centralized and standardized (using the “scale” function in R). The formula for calculating the risk score was as follows: (X: coefficients, Y: lncRNA expression level). The median risk score was used to separate the TCGA THCA patients into low- and high-risk subgroups, and Kaplan-Meier analysis was utilized to compare the PFS of the two groups. The predictive value of the m6A-lncRNAs was evaluated using the areas under the curve (AUCs) of receiver operating characteristic (ROC) curves.
RT-qPCR
For the detection of m6A-related lncRNA expression, Trizol Reagent (Invitrogen, Carlsbad, CA, USA) was utilized for extraction of total RNA from THCA tissues, and RT Reagent Kit with gDNA Eraser (TaKaRa Bio Inc., Kusatsu, Shiga, Japan) was utilized for reverse transcription. SYBR-Green (TaKaRa Bio Inc.) and RT-qPCR analysis were then used to detect complementary DNA (cDNA) expression levels using glyceraldehyde-3-phosphate dehydrogenase (GAPDH) as the internal reference. The primers are detailed in Table 1. PCR amplification was done in a 3-well format. Each experiment was carried out 3 times and the relative expression levels of genes were determined with 2−ΔΔCt.
Table 1
Gene | Primers |
---|---|
CTA-398F10.2 | F: 5'-gcaggcttaagcacatctcc-3' |
R: 5'-gcggtgtttggttttctgtt-3' | |
AC008992.1 | F: 5'-atcccaagcaggtgaacaag-3' |
R: 5'-gtgggtgcattcaatcagaa-3' | |
AC004160.4 | F: 5'-cagctttgacccctgtgatt-3' |
R: 5'-aggggtttttcaaaggcagt-3' | |
AC083843.2 | F: 5'-ctcagtctccaagccctgtc-3' |
R: 5'-atagcttcagggtggggtct-3' | |
MORC2-AS1 | F: 5'-ctcaccacagatcagccaga-3' |
R: 5'-aaaatgcaggggtcctcttt-3' | |
RP11-409I10.2 | F: 5'-tgtcaaaggcaaaatgctga-3' |
R: 5'-catgttcaatgcgatgttcc-3' | |
AC011298.2 | F: 5'-gacatggcttcaagtcagca-3' |
R: 5'-aatgggaccacgagctacac-3' | |
RP11-395N3.1 | F: 5'-ggtgaaaactccatgccact-3' |
R: 5'-ccccaaatcaccaatcaatc-3' | |
β-actin | F: 5'-cctctcccaagtccacacag-3' |
R: 5'-gggcacgaaggctcatcatt-3' | |
GAPDH | F: 5'-aaggtgaaggtcggagtcaac-3' |
R: 5'-ggggtcattgatggcaacaata-3' |
RT-qPCR, real-time quantitative polymerase chain reaction; GAPDH, glyceraldehyde-3-phosphate dehydrogenase; F, forward; R, reverse.
Statistical analysis
Statistical analyses were carried out using R software (version 4.1.1) and executed with SPSS 21.0 (IBM Corp., Armonk, NY, USA). To determine statistically significant differences, Student’s t-test or one-way analysis of variance (ANOVA) with Tukey’s multiple comparison post-hoc test were used. Each independent experiment was performed at least 3 times. A value of P<0.05 was considered a statistically significant difference.
Results
LncRNAs associated with recurrence of thyroid carcinoma
We analyzed THCA and normal tissue groups for DELs (log2fold change >1 and P<0.05) based on 10,609 lncRNAs. As shown in the volcano plots, we identified 697 DELs, including 220 upregulated and 477 downregulated DELs (Figure 1A). Differential expression analysis of lncRNAs related to recurrence of THCA was performed using the limma package in R. As a result, 645 DELs were detected, of which 118 were upregulated and 527 were downregulated (Figure 1B). The data of the above 2 results were intersected to obtain 8 lncRNAs strongly associated with recurrence of THCA (Figure 1C). The expression levels of the 8 lncRNAs were shown to have an overall positive correlation (Figure 1D).
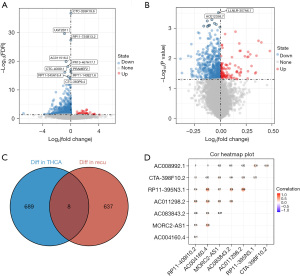
Coexpression network of m6A-related lncRNAs in recurrence of THCA
There are 23 human m6A genes, including 8 writers, 13 readers, and 2 erasers. Their expression levels in THCA and normal tissues adjacent to the cancer are shown in Figure 2A. Only IGFBP1 showed low expression, while the other m6A genes were highly expressed. In addition, we further analyzed the differential expression of 23 m6A genes between recurrent and nonrecurrent THCA samples. As shown in Figure 2B, only YTHDF3 expression was significant (P<0.05), and the expression differences of the remaining 22 m6A genes were not significant between recurrent and nonrecurrent THCA samples.
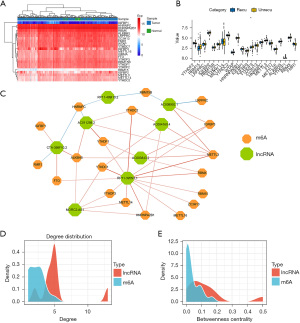
In order to construct a m6A-lncRNA coexpression network related to THCA recurrence [m6A-lncRNA coexpression network in recurrence (MLCR)], we performed Pearson correlation analysis of the expression levels of lncRNAs as well as m6A genes, and 19 m6A genes were screened from 23 m6A genes, with 8 lncRNAs forming a m6A-lncRNA coexpression network associated with THCA recurrence (Figure 2C). The network contained 43 gene pairs and 27 nodes, including 8 lncRNAs and 19 m6A genes. The node degree distribution and betweenness centrality of m6A and lncRNAs in the m6A-lncRNA coexpression network are shown in Figure 2D,2E, respectively. We could conclude that the node degree distribution of lncRNAs was more concentrated than m6A. These results indicated that 1 lncRNA could regulate more than 1 m6A gene at the same time. The node degree distribution and the betweenness centrality of lncRNAs were generally higher than that of m6A. The results also showed that lncRNAs had stronger regulatory effects on m6A.
Clustering analysis of m6A-related recurrent lncRNAs
Based on the expression levels of the 8 lncRNAs, we identified distinct subgroups among 108 tumor samples by k-means consensus-cluster analysis. In the TCGA-THCA dataset, the cumulative distribution function (CDF) curve showed cluster stability rising from k=2 to 9 (Figure 3A). As depicted in Figure 3B, k=3 was a reasonable choice, and THCA patients were clustered into 3 distinct clusters. We further explored the correlation between THCA clusters and clinical characteristics, including recurrence, stage, age, gender, and TNM. THCA patients in cluster 1 tended to have higher recurrence rates compared to patients in cluster 2 and 3 (Figure 3C). In summary, consensus clustering analysis showed that cluster 1 may be associated with THCA recurrence.
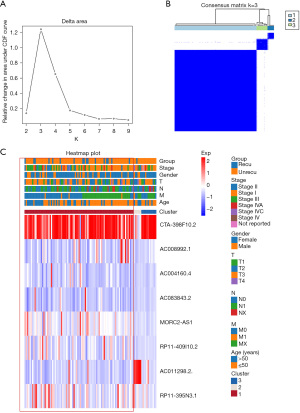
Immunoassay and pathway enrichment analysis of THCA clusters
To understand the effect of tumor-infiltrating immune cells on THCA recurrence, the composition of significant tumor-infiltrating immune cells was assessed for the 3 THCA clusters using the CIBERSORT algorithm. Numbers of resting memory CD4 T cells, M0 macrophages, and M2 macrophages were higher in cluster 1 than 3 (Figure 4A). Compared with cluster 2 and 3, the number of B cells was significantly higher in cluster 1 (Figure 4B), while the number of CD8 T cells was slightly lower in cluster 1 (Figure 4C). Moreover, the immune and stromal scores were significantly increased in cluster 1 compared to cluster 3, while compared with cluster 2, the opposite results were observed (Figure 4D,4E). Taken together, these results revealed that m6A-related recurrent lncRNAs were closely associated with B cells.
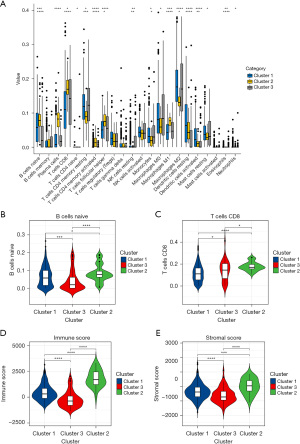
In order to further clarify the potential functions of the 3 clusters in THCA, Kyoto Encyclopedia of Genes and Genomes (KEGG) pathway analysis was conducted to compare cluster 1 and cluster 2 or cluster 3 using GSEA software. Butyrate metabolism, regulation of actin cytoskeleton, apoptosis, cell adhesion molecules, leukocyte transendothelial migration, and natural killer cell-mediated cytotoxicity were dramatically enriched in cluster 1 and cluster 2 (Figure 5A). Butyrate metabolism, autoimmune thyroid disease, viral myocarditis, natural killer cell-mediated cytotoxicity, antigen processing and presentation, and cell adhesion molecules were significantly enriched in cluster 1 and cluster 3 (Figure 5B). Three pathways were significant in both of the above pathway analyses, namely natural killer cell-mediated cytotoxicity, cell adhesion molecules, and butyrate metabolism.
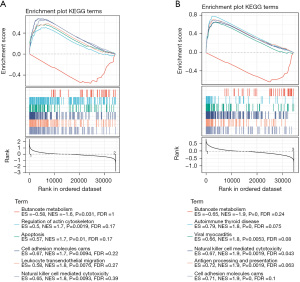
Survival analysis and validation of 8 m6A-related lncRNAs in recurrent THCA
In order to investigate the prognostic value of m6A-related lncRNAs in THCA, PFS data for THCA were downloaded from the TCGA Pan-Cancer Clinical Data Resource (18), and survival analysis was performed for the 8 screened m6A-related lncRNAs associated with THCA recurrence. We then constructed a risk model of 8 m6A-related lncRNAs. Based on the median risk score value, 108 THCA patients with complete survival time and status data were categorized into either a high-risk group (n=54) or low-risk group (n=54) (Figure 6A, upper). Survival analysis of risk groups indicated that THCA cases in the high-risk group had a greater probability of progression than those in the low-risk group (Figure 6A, middle). The relative expression levels of the 8 m6A-related lncRNAs for each patient are shown in Figure 6A (bottom). To assess the specificity and sensitivity of the m6A-lncRNA model, we performed ROC curve analysis. The m6A-related lncRNAs were found to have a high level of efficacy in predicting PFS of recurrent THCA patients. The AUCs of the ROC curves for 1-, 3-, and 5-year survival were 0.69, 0.79, and 0.81, respectively (Figure 6B). Survival analysis was carried out for the two risk groups. We discovered that being in the high-risk group was linked to an unfavorable prognosis in THCA (P<0.0001, Figure 6C). Overall, these results suggested that m6A-related lncRNAs had a good ability to predict survival in THCA patients. Together, these data revealed the accuracy of the risk model of the selected genes for THCA prognosis.
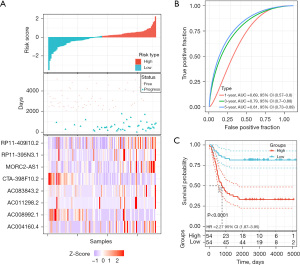
Risk score model can be applied as a predictor of THCA recurrence
The validation cohort was designed to verify the risk score in predicting recurrence of THCA. A total of 200 THCA patient samples were collected, including 20 recurrent samples. In addition, we randomly selected 20 from the remaining 180 nonrecurrent samples as control samples. The expression levels of 8 m6A-related lncRNAs were detected by RT-qPCR in the selected 40 patient samples (Figure 7A). The results were then substituted into the risk-score formula to obtain the risk score values. We performed statistical analysis based on the risk score values of the THCA.
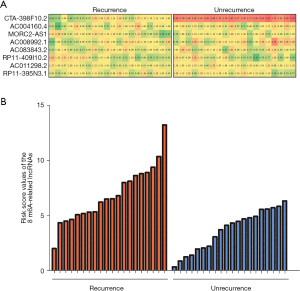
Risk score = exp(CTA-398F10.2) × −1.23 + exp(AC004160.4) × −0.52 + exp(MORC2-AS1) × 4.4 + exp(AC008992.1) × −2.93 + exp(AC083843.2) × 1.55 + exp(RP11-409I10.2) × 4.46 + exp(AC011298.2) × −0.03 + exp(RP11-395N3.1) × 0.991.
As shown in Figure 7B, the risk assessment score was significantly higher in the recurrence group compared with the unrecurrence group, indicating that the expression levels of the 8 m6A-related lncRNAs had a high predictive value for recurrence in THCA patients. The above experimental results were consistent with the results of previous bioinformatic analysis.
Discussion
THCA is one of the most frequent cancers. Although the prognosis is usually good, many patients still have recurrence after treatment (19). Prognostic tools such as the MACIS score and the American Joint Committee on Cancer (AJCC) staging system focus on clinical features only, making it difficult to accurately estimate the specific risk of recurrence for individual patients (20,21). As epigenetics and molecular biology have developed, novel m6A methylation modifications have received increasing attention (22,23). M6A has been found to be the most significant and abundant posttranscriptional modification in eukaryotic RNA. Most of the genes modified by m6A are oncogenes. This modification will lead to changes in mRNA translation, thus accelerating the progress of tumors. It has been reported that m6A demethylase FTO is a prognostic marker of non-squamous cell carcinoma and promotes the proliferation and invasion of cancer cells. Methyltransferase 14 can promote the development of hepatocellular carcinoma by regulating m6A dependent microRNA (miRNA). These indicate that m6A plays a crucial role in multiple tumor types via a variety of mechanisms (24,25). The existing prognostic markers of THCA are mainly the combination of genetic factors and overexpression proteins. Genetic markers include mutations of RAS, BRAF and TERT oncogenes. Overexpression of proteins is mainly LGALS3 and FOXP3. But these biomarkers are not very reliable. For example, BRAF mutation is regarded as a prognostic marker related to recurrence, but in another study, it has little prognostic value in PTC. It can be seen that more biomarkers are needed to jointly judge the prognosis of cancer. The recurrence of THCA is mainly related to epithelial mesenchymal transformation (EMT). Therefore, the lncRNA that recognizes EMT may be a marker for early detection of cancer recurrence (26). LncRNAs are receiving increasing attention as a class of tumor biomarkers for early screening, targeted therapy, and prognostic evaluation (27). There is mounting evidence that lncRNAs are closely associated with the recurrence of tumors (28,29). Here, we found that m6A-lncRNA may be associated with THCA recurrence. Although current prognostic tools for THCA have been investigated, integrating multiple biomarkers into a single model could improve current guidelines and provide clinicians with more accurate judgements, regardless of age, tumor size, and stage.
In the current study, we obtained 58 paracancer controls and 510 THCA samples from the TCGA dataset, the differentially expressed genes were obtained by analyzing the RNA seq expression data. Then 108 recurrent and non-recurrent samples containing clinical data were analyzed to obtain the recurrence related differential expression of lncRNAs. Two types of differentially expressed genes intersect, and ultimately screened 8 lncRNAs that were strongly associated with THCA recurrence. Because the methylation modification of m6A may affect the noncoding RNA, thereby affecting the prognosis of cancer. We performed Pearson analysis on relapse related lncRNAs and m6A-related genes, and the results showed that these 8 lncRNAs were also strongly related with m6A. Based on these results, we constructed a m6A-lncRNA coexpression network for THCA recurrence. On the basis of the expression levels of the 8 m6A-related lncRNAs, we used k-means clustering analysis on the THCA samples in the TCGA dataset and obtained 3 clusters. Subsequent correlation analysis between the clustering analysis and other clinical parameters showed that cluster 1 was most associated with recurrence of THCA, which was further validated by RT-qPCR for the 8 m6A-related lncRNAs in recurrent versus nonrecurrent THCA. The above results revealed that the 8 m6A-related lncRNAs could have promising clinical application as a detection model for predicting THCA recurrence.
THCA tissue contains both malignant and normal cells, inclusive of immune cells and stromal cells (30,31). These cells have evolved into a complicated whole that generates complex signals for communication (32,33). We established a tumor immune infiltrating cell expression profile for THCA clusters using the THCA transcriptome sequencing data and clinical features obtained by TCGA-THCA. In THCA, the immunological microenvironment is exceedingly heterogeneous, with large differences in the number of cells of various types. In this study, we detected significantly lower levels of CD8 T cells and follicular helper T cells in cluster 1, which was associated with THCA recurrence, than in clusters 2 and 3, while the levels of dendritic cells (DCs), M2 macrophages, resting DCs, regulatory T cells, B cells and resting mast cells were dramatically higher in cluster 1 than in clusters 2 and 3. CD8+ T cells, a major part of the immune system, are often incorrectly called cytotoxic T lymphocytes (CTLs) or killer T cells (34). It has been suggested that a decrease in CD8 T cells is associated with tumor progression and prognosis of recurrence. DCs in tumors have an antigen-presenting and activating role and directly activate T cells, which have a constantly renewed function in vivo and can be involved in multiple dynamic phases or functional subclasses at the same time (35,36). Diverse T-cell subsets play different roles in the immune response, such as releasing lymphokines, killing target cells, aiding the immune response, and triggering memory-specific antigens (37). The differences in immune and stromal scores between the recurrence and unrecurrence THCA groups were statistically significant. In general, the changes of immune microenvironment can be used as a reference for THCA recurrence, especially the significant decrease of CD8 T cell level and the significant increase of B cell level in recurrence related cluster 1. Cluster 1 was obtained by cluster analysis based on m6A-related lncRNAs expression levels. This also shows that m6A-related lncRNAs can affect the level changes of immune cells in the immune microenvironment. At the same time, these m6A-related lncRNAs can be used as a detection model to predict THCA recurrence, providing a new direction for the prognosis diagnosis of THCA.
Our study had some limitations. First, the study was based on a public dataset and lncRNA-related prognostic model, which we tested using 40 THCA patient samples from our center. However, the number of verified samples was small and should be enlarged in future work. Next, the different types of THCA usually present with molecular and pathologic differences. However, we analyzed the data of the whole THCA patient group and not specific types of THCA because of the small number of patient samples. Third, although our study constructed a prediction model based on 8 associated lncRNAs, the mechanisms of these lncRNAs in regulating THCA progression should be explored in future studies.
Conclusions
In view of the above findings, we could conclude that increased tumor infiltrating cells and dysregulation of immune cells played an essential role in the recurrence of THCA. Enrichment pathway analysis revealed shared pathway enrichment for natural killer cell-mediated cytotoxicity, cell adhesion molecules, and butyrate metabolism. We found the m6A-related lncRNA risk model was more effective in predicting PFS in patients with THCA recurrence.
Acknowledgments
Funding: This study was funded by the Scientific Research Project of Heilongjiang Municipal Commission of Health and Family Planning (No. 2020-328).
Footnote
Reporting Checklist: The authors have completed the TRIPOD reporting checklist. Available at https://gs.amegroups.com/article/view/10.21037/gs-22-678/rc
Data Sharing Statement: Available at https://gs.amegroups.com/article/view/10.21037/gs-22-678/dss
Conflicts of Interest: All authors have completed the ICMJE uniform disclosure form (available at https://gs.amegroups.com/article/view/10.21037/gs-22-678/coif). All authors report that this study was funded by the Scientific Research Project of Heilongjiang Municipal Commission of Health and Family Planning (No. 2020- 328). The authors have no other conflicts of interest to declare.
Ethical Statement: The authors are accountable for all aspects of the work in ensuring that questions related to the accuracy or integrity of any part of the work are appropriately investigated and resolved. The study was conducted in accordance with the Declaration of Helsinki (as revised in 2013). The study was approved by Medical Ethics Committee of the First Affiliated Hospital of Jiamusi University (No. 2020023) and informed consent was taken from all the patients.
Open Access Statement: This is an Open Access article distributed in accordance with the Creative Commons Attribution-NonCommercial-NoDerivs 4.0 International License (CC BY-NC-ND 4.0), which permits the non-commercial replication and distribution of the article with the strict proviso that no changes or edits are made and the original work is properly cited (including links to both the formal publication through the relevant DOI and the license). See: https://creativecommons.org/licenses/by-nc-nd/4.0/.
References
- Howlader N, Noone AM, Krapcho M. SEER Cancer Statistics Review, 1975-2014. Bethesda: National Cancer Institute, 2017.
- Cheng Q, Li X, Acharya CR, et al. A novel integrative risk index of papillary thyroid cancer progression combining genomic alterations and clinical factors. Oncotarget 2017;8:16690-703. [Crossref] [PubMed]
- Santiago K, Chen Wongworawat Y, Khan S. Differential MicroRNA-Signatures in Thyroid Cancer Subtypes. J Oncol 2020;2020:2052396. [Crossref] [PubMed]
- Pontius LN, Youngwirth LM, Thomas SM, et al. Lymphovascular invasion is associated with survival for papillary thyroid cancer. Endocr Relat Cancer 2016;23:555-62. [Crossref] [PubMed]
- Ge MH, Cao J, Wang JY, et al. Nomograms predicting disease-specific regional recurrence and distant recurrence of papillary thyroid carcinoma following partial or total thyroidectomy. Medicine (Baltimore) 2017;96:e7575. [Crossref] [PubMed]
- Zhang D, Wu M, Xiong M, et al. Long Noncoding RNAs: An Overview. Methods Mol Biol 2021;2372:297-305. [Crossref] [PubMed]
- Camacho CV, Choudhari R, Gadad SS. Long noncoding RNAs and cancer, an overview. Steroids 2018;133:93-5. [Crossref] [PubMed]
- Choudhari R, Sedano MJ, Harrison AL, et al. Long noncoding RNAs in cancer: From discovery to therapeutic targets. Adv Clin Chem 2020;95:105-47. [Crossref] [PubMed]
- Lu W, Cao F, Wang S, et al. LncRNAs: The Regulator of Glucose and Lipid Metabolism in Tumor Cells. Front Oncol 2019;9:1099. [Crossref] [PubMed]
- Zhou Y, Zhu Y, Xie Y, et al. The Role of Long Noncoding RNAs in Immunotherapy Resistance. Front Oncol 2019;9:1292. [Crossref] [PubMed]
- Liu Y, Li J, Li F, et al. SNHG15 functions as a tumor suppressor in thyroid cancer. J Cell Biochem 2019;120:6120-6. [Crossref] [PubMed]
- Dai F, Wu Y, Lu Y, et al. Crosstalk between RNA m6A Modification and Non-coding RNA Contributes to Cancer Growth and Progression. Mol Ther Nucleic Acids 2020;22:62-71. [Crossref] [PubMed]
- Chen C, Guo Y, Guo Y, et al. m6A Modification in NonCoding RNA: The Role in Cancer Drug Resistance. Front Oncol 2021;11:746789. [Crossref] [PubMed]
- Patil DP, Chen CK, Pickering BF, et al. m(6)A RNA methylation promotes XIST-mediated transcriptional repression. Nature 2016;537:369-73. [Crossref] [PubMed]
- Yang F, Jin H, Que B, et al. Dynamic m6A mRNA methylation reveals the role of METTL3-m6A-CDCP1 signaling axis in chemical carcinogenesis. Oncogene 2019;38:4755-72. [Crossref] [PubMed]
- Choe J, Lin S, Zhang W, et al. mRNA circularization by METTL3-eIF3h enhances translation and promotes oncogenesis. Nature 2018;561:556-60. [Crossref] [PubMed]
- Yu X, Dong P, Yan Y, et al. Identification of N6-Methyladenosine-Associated Long Non-coding RNAs for Immunotherapeutic Response and Prognosis in Patients With Pancreatic Cancer. Front Cell Dev Biol 2021;9:748442. [Crossref] [PubMed]
- Liu J, Lichtenberg T, Hoadley KA, et al. An Integrated TCGA Pan-Cancer Clinical Data Resource to Drive High-Quality Survival Outcome Analytics. Cell 2018;173:400-16.e11. [Crossref] [PubMed]
- Haugen BR, Alexander EK, Bible KC, et al. 2015 American Thyroid Association Management Guidelines for Adult Patients with Thyroid Nodules and Differentiated Thyroid Cancer: The American Thyroid Association Guidelines Task Force on Thyroid Nodules and Differentiated Thyroid Cancer. Thyroid 2016;26:1-133. [Crossref] [PubMed]
- Carty SE, Doherty GM, Inabnet WB 3rd, et al. American Thyroid Association statement on the essential elements of interdisciplinary communication of perioperative information for patients undergoing thyroid cancer surgery. Thyroid 2012;22:395-9. [Crossref] [PubMed]
- Passler C, Prager G, Scheuba C, et al. Application of staging systems for differentiated thyroid carcinoma in an endemic goiter region with iodine substitution. Ann Surg 2003;237:227-34. [Crossref] [PubMed]
- Hou P, Meng S, Li M, et al. LINC00460/DHX9/IGF2BP2 complex promotes colorectal cancer proliferation and metastasis by mediating HMGA1 mRNA stability depending on m6A modification. J Exp Clin Cancer Res 2021;40:52. [Crossref] [PubMed]
- Hu Y, Ouyang Z, Sui X, et al. Oocyte competence is maintained by m6A methyltransferase KIAA1429-mediated RNA metabolism during mouse follicular development. Cell Death Differ 2020;27:2468-83. [Crossref] [PubMed]
- Patil DP, Pickering BF, Jaffrey SR. Reading m6A in the Transcriptome: m6A-Binding Proteins. Trends Cell Biol 2018;28:113-27. [Crossref] [PubMed]
- Sun T, Wu R, Ming L. The role of m6A RNA methylation in cancer. Biomed Pharmacother 2019;112:108613. [Crossref] [PubMed]
- Zhao H, De Souza C, Kumar VE, et al. Long noncoding RNA signatures as predictors of prognosis in thyroid cancer: a narrative review. Ann Transl Med 2021;9:359. [Crossref] [PubMed]
- Melaccio A, Sgaramella LI, Pasculli A, et al. Prognostic and Therapeutic Role of Angiogenic Microenvironment in Thyroid Cancer. Cancers (Basel) 2021;13:2775. [Crossref] [PubMed]
- Li P, Duan S, Fu A. Long noncoding RNA NEAT1 correlates with higher disease risk, worse disease condition, decreased miR-124 and miR-125a and predicts poor recurrence-free survival of acute ischemic stroke. J Clin Lab Anal 2020;34:e23056. [Crossref] [PubMed]
- Wang K, Li J, Xiong YF, et al. A Potential Prognostic Long Noncoding RNA Signature to Predict Recurrence among ER-positive Breast Cancer Patients Treated with Tamoxifen. Sci Rep 2018;8:3179. [Crossref] [PubMed]
- Chen YP, Zhang J, Wang YQ, et al. The immune molecular landscape of the B7 and TNFR immunoregulatory ligand-receptor families in head and neck cancer: A comprehensive overview and the immunotherapeutic implications. Oncoimmunology 2017;6:e1288329. [Crossref] [PubMed]
- Mandal R, Şenbabaoğlu Y, Desrichard A, et al. The head and neck cancer immune landscape and its immunotherapeutic implications. JCI Insight 2016;1:e89829. [Crossref] [PubMed]
- Rui X, Shao S, Wang L, et al. Identification of recurrence marker associated with immune infiltration in prostate cancer with radical resection and build prognostic nomogram. BMC Cancer 2019;19:1179. [Crossref] [PubMed]
- Jiang B, Sun Q, Tong Y, et al. An immune-related gene signature predicts prognosis of gastric cancer. Medicine (Baltimore) 2019;98:e16273. [Crossref] [PubMed]
- Morvan MG, Teque FC, Locher CP, et al. The CD8+ T Cell Noncytotoxic Antiviral Responses. Microbiol Mol Biol Rev 2021;85:e00155-20. [Crossref] [PubMed]
- Ge P, Wang W, Li L, et al. Profiles of immune cell infiltration and immune-related genes in the tumor microenvironment of colorectal cancer. Biomed Pharmacother 2019;118:109228. [Crossref] [PubMed]
- Yang S, Liu T, Cheng Y, et al. Immune cell infiltration as a biomarker for the diagnosis and prognosis of digestive system cancer. Cancer Sci 2019;110:3639-49. [Crossref] [PubMed]
- Hao Z, Guo D. EGFR mutation: novel prognostic factor associated with immune infiltration in lower-grade glioma; an exploratory study. BMC Cancer 2019;19:1184. [Crossref] [PubMed]
(English Language Editor: A. Muijlwijk)